
When first encountered, this may not sound like a good idea. That’s the process of filling in missing data using a best-estimate from all the other data that exists.
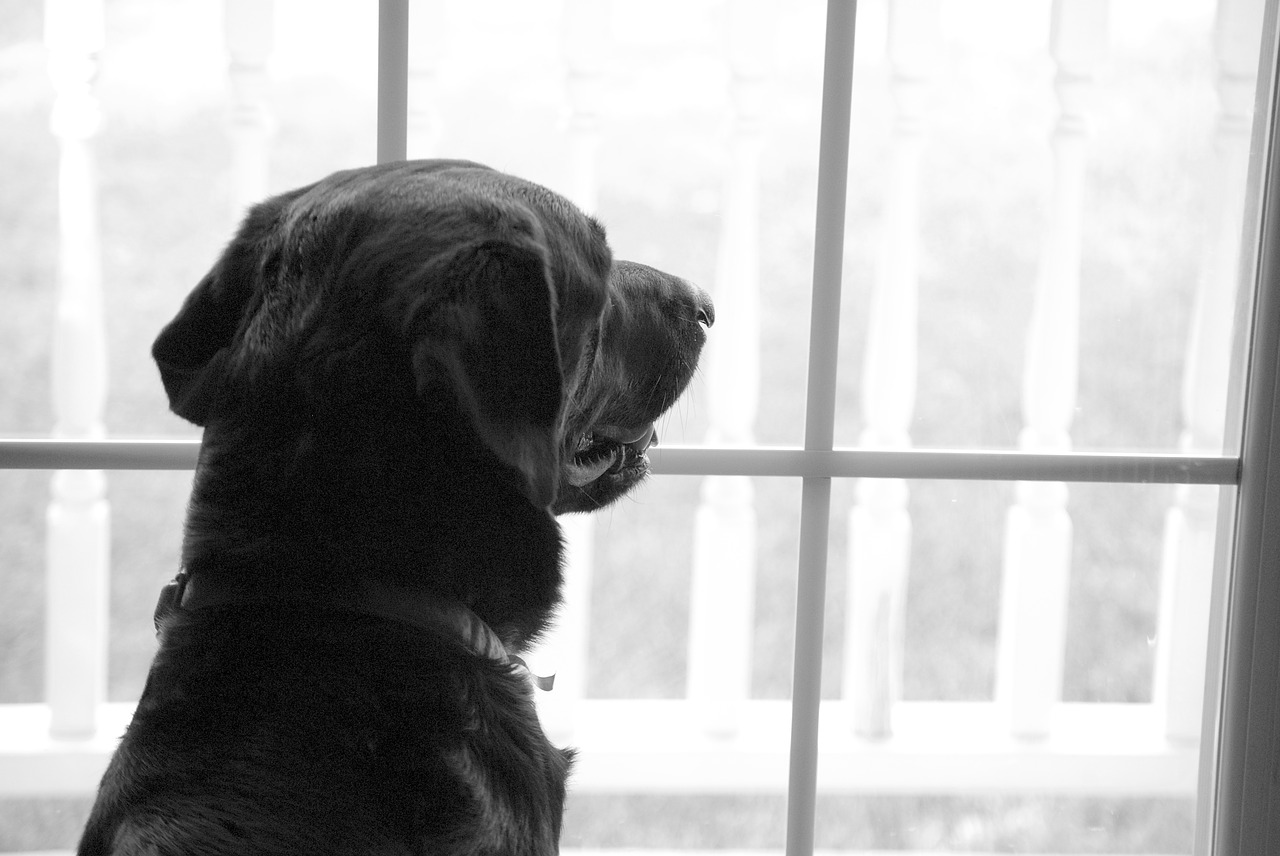
Mice is our go to package for multiple imputation. Pass either or neither e.g. to summarise data frame or tibble:Įxplanatory = c( "age", "sex.factor", "nodes", "obstruct.factor", "smoking_mcar") dependent = "mort_5yr" colon_s %>% finalfit(dependent, explanatory) %>% knitr :: kable( row.names= FALSE, align = c( "l", "l", "r", "r", "r", "r")) # Omit when you run #> Note: dependent includes missing data. The dependent and explanatory are for convenience. The function summarises a data frame or tibble by numeric (continuous) variables and factor (discrete) variables. Library(finalfit) # Create some extra missing data # Smoking missing completely at random set.seed( 1) colon_s $smoking_mcar = sample( c( "Smoker", "Non-smoker", NA), dim(colon_s), replace= TRUE, prob = c( 0.2, 0.7, 0.1)) %>% factor() %>% ff_label( "Smoking (MCAR)") # Smoking missing conditional on patient sex colon_s $smoking_mar = sample( c( "Smoker", "Non-smoker", NA), sum(colon_s $sex.factor = "Female"), replace = TRUE, prob = c( 0.1, 0.5, 0.4)) colon_s $smoking_mar = sample( c( "Smoker", "Non-smoker", NA), sum(colon_s $sex.factor = "Male"), replace= TRUE, prob = c( 0.15, 0.75, 0.1)) colon_s $smoking_mar = factor(colon_s $smoking_mar) %>% ff_label( "Smoking (MAR)") # Examine with ff_glimpse explanatory = c( "age", "sex.factor", "nodes", "obstruct.factor", "smoking_mcar", "smoking_mar") dependent = "mort_5yr" colon_s %>% ff_glimpse(dependent, explanatory) #> $Continuous #> label var_type n missing_n missing_percent mean sd min #> age Age (years) 929 0 0.0 59.8 11.9 18.0 #> nodes nodes 911 18 1.9 3.7 3.6 0.0 #> quartile_25 median quartile_75 max #> age 53.0 61.0 69.0 85.0 #> nodes 1.0 2.0 5.0 33.0 #> #> $Categorical #> label var_type n missing_n missing_percent #> mort_5yr Mortality 5 year 915 14 1.5 #> sex.factor Sex 929 0 0.0 #> obstruct.factor Obstruction 908 21 2.3 #> smoking_mcar Smoking (MCAR) 828 101 10.9 #> smoking_mar Smoking (MAR) 719 210 22.6 #> levels_n levels levels_count #> mort_5yr 2 "Alive", "Died", "(Missing)" 511, 404, 14 #> sex.factor 2 "Female", "Male" 445, 484 #> obstruct.factor 2 "No", "Yes", "(Missing)" 732, 176, 21 #> smoking_mcar 2 "Non-smoker", "Smoker", "(Missing)" 645, 183, 101 #> smoking_mar 2 "Non-smoker", "Smoker", "(Missing)" 591, 128, 210 #> levels_percent #> mort_5yr 55.0, 43.5, 1.5 #> sex.factor 48, 52 #> obstruct.factor 78.8, 18.9, 2.3 #> smoking_mcar 69, 20, 11 #> smoking_mar 64, 14, 23
